Deepfake
Deepfake, a portmanteau of “deep learning” and “fake”, is the utilisation of artificial intelligence (AI) / deep learning algorithms to create believable or realistic videos, images and audios which never happen. An example of its application is to modify film footage instead of re-shooting and refining advanced face attributes in post-production.
In recent years, deepfakes have attracted public attention for their malicious uses in the creation of fake videos, forged images and financial fraud, resulting in the spread of misinformation or disinformation which can potentially erode the reputation of businesses and trust among people. Nowadays, tools that create deepfakes are becoming more readily available. Plausible deepfakes have elicited public responses to detect and limit their use.
Technology to create such synthetic data keeps evolving over time.
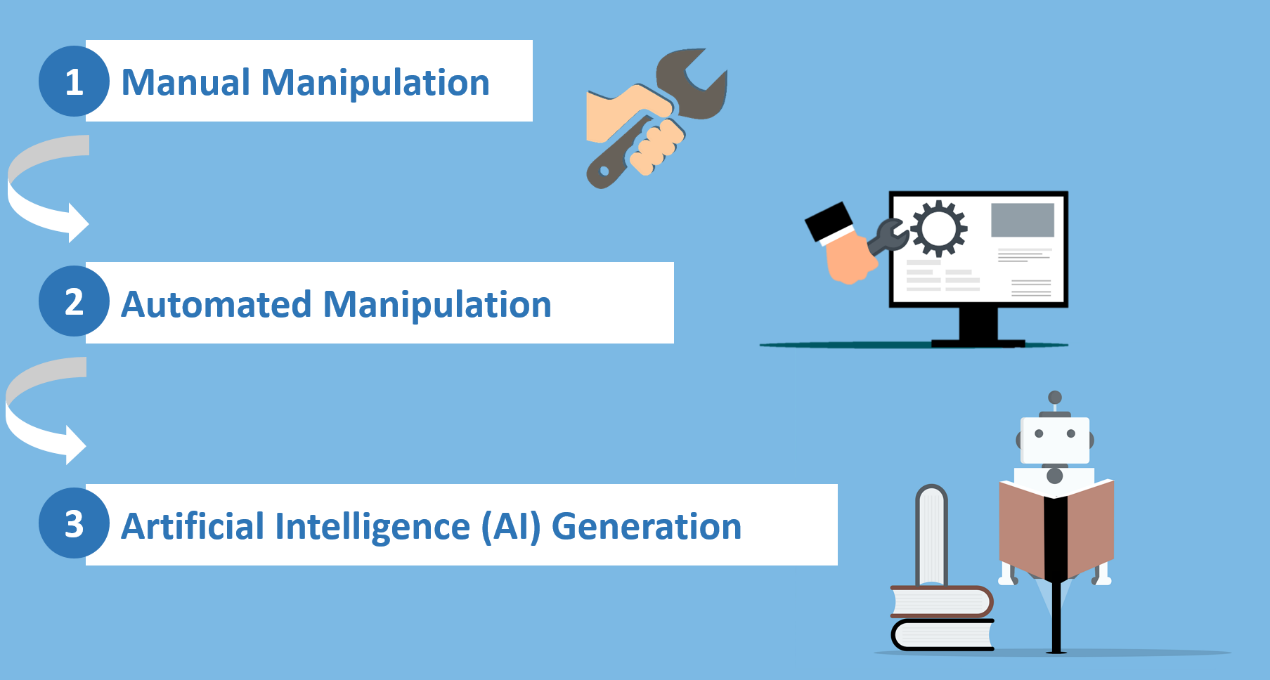
Figure 1: Evolution of Deepfake
Deepfake technology includes a list of algorithms to recognise the most important parameters of human faces and voices, and learn to reconstruct them in synthetic videos, images and audios. The technologies are broadly categorised into visual and audio groups, and attributes manipulation levels are classified by source media and synthetic result is required.
The audio-based deepfake technique is used to create a convincing speech that sounds like given by a particular person who actually has not said so.
The visual-based deepfake technique is used to create falsified videos which a person in an existing image or video is replaced with someone else’s likeness.
Since the quality of deepfakes is increasingly realistic and convincing, people can hardly distinguish fake attributes from a real video. Deepfakes seem so real that the viewers are led to believe that they are real and share them on social media channels thus hastening the spread of disinformation.
Many malicious deepfakes have been identified and some of the impacts of deepfakes are listed below.
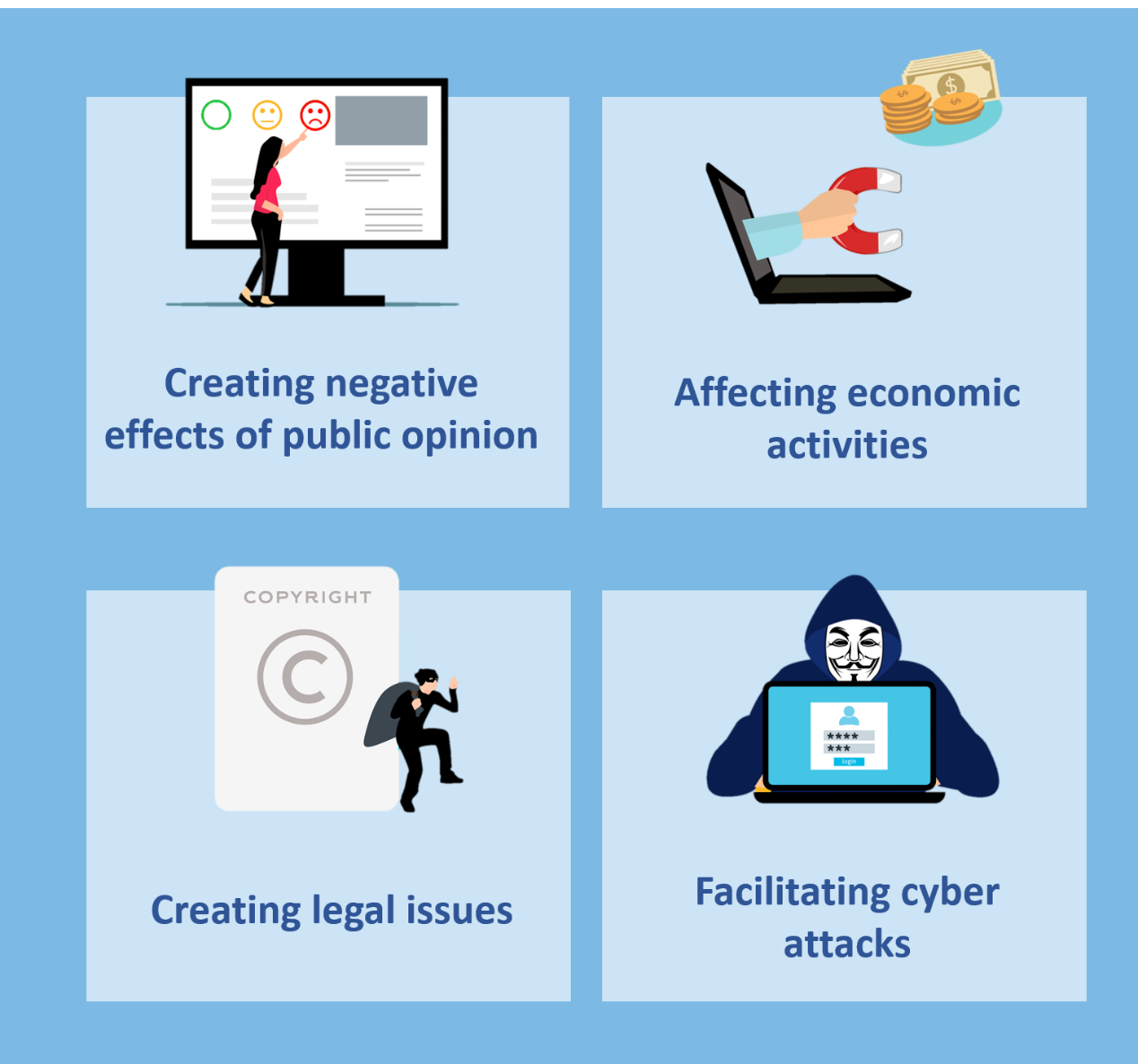
Figure 2: Examples of Deepfake Impacts
Cause serious damage to victim's reputation such as misrepresenting certain celebrities using their faces or voices to spread rumours.
Depict make-up events to defame enterprises which may in turn drive the sentiments of the market and cause turmoil on stock market.
Deepfakes may involve reproducing and distributing copyright material without owners’ permission and improper use may lead to legal problems. Similarly, disclosing or altering someone’ personal information may become subjects of privacy breaches. For litigation in court, dispute concerning electronic evidences such as audio or video recordings can be used only if its authenticity is testified by forensics watermarking tools.
Deepfakes may have significant potential to enhance cyber attacks and scams.
With the wide application of deepfake technology, it is increasingly difficult to believe what we see or hear. Thus, the detection of deepfakes is crucial to the protection of people’s trust in the digital content. Most of the work in detecting deepfakes make use of deep learning methods and exploiting visual artifacts present in the face regions. By recognising patterns in how deepfakes are created, it is able to pick up subtle inconsistencies using deep learning. Apart from using technologies, there are some general tips for detecting deepfakes manually:
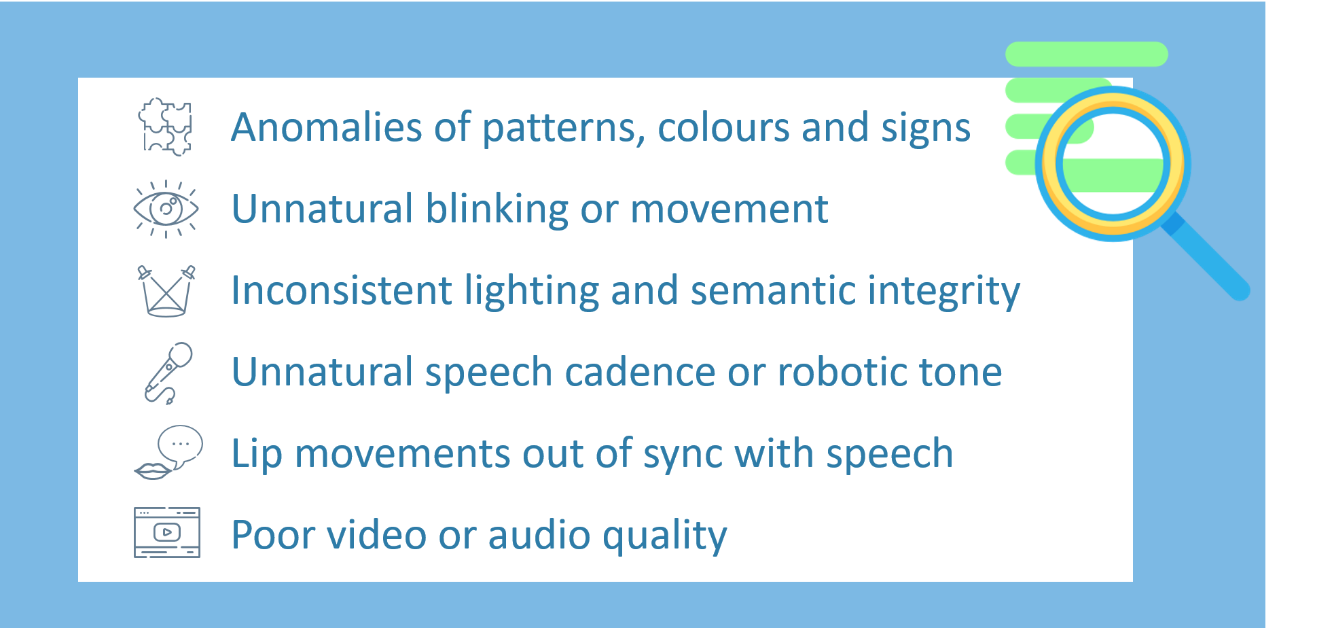
Figure 3: General Tips for Detecting Deepfakes
Given the threats posed by deepfake technology carry serious and far-reaching implications for business, it is important to take preventive measures to reduce the chance of falling prey to deepfake-based frauds.
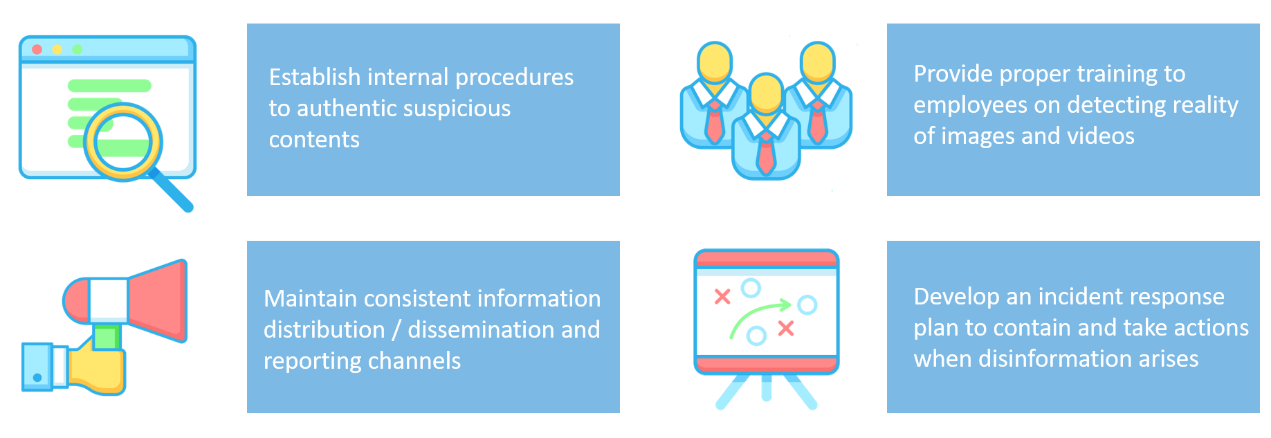
Figure 4: Best Practices for Organisations
Disclaimer: Users are also recommended to observe the disclaimer of this website and read the user agreements and privacy policies of the security software and tools before downloading and using them.